The Power of AI and ML in Modern Network Traffic Analysis
Gusztáv Krékity
2025.04.11
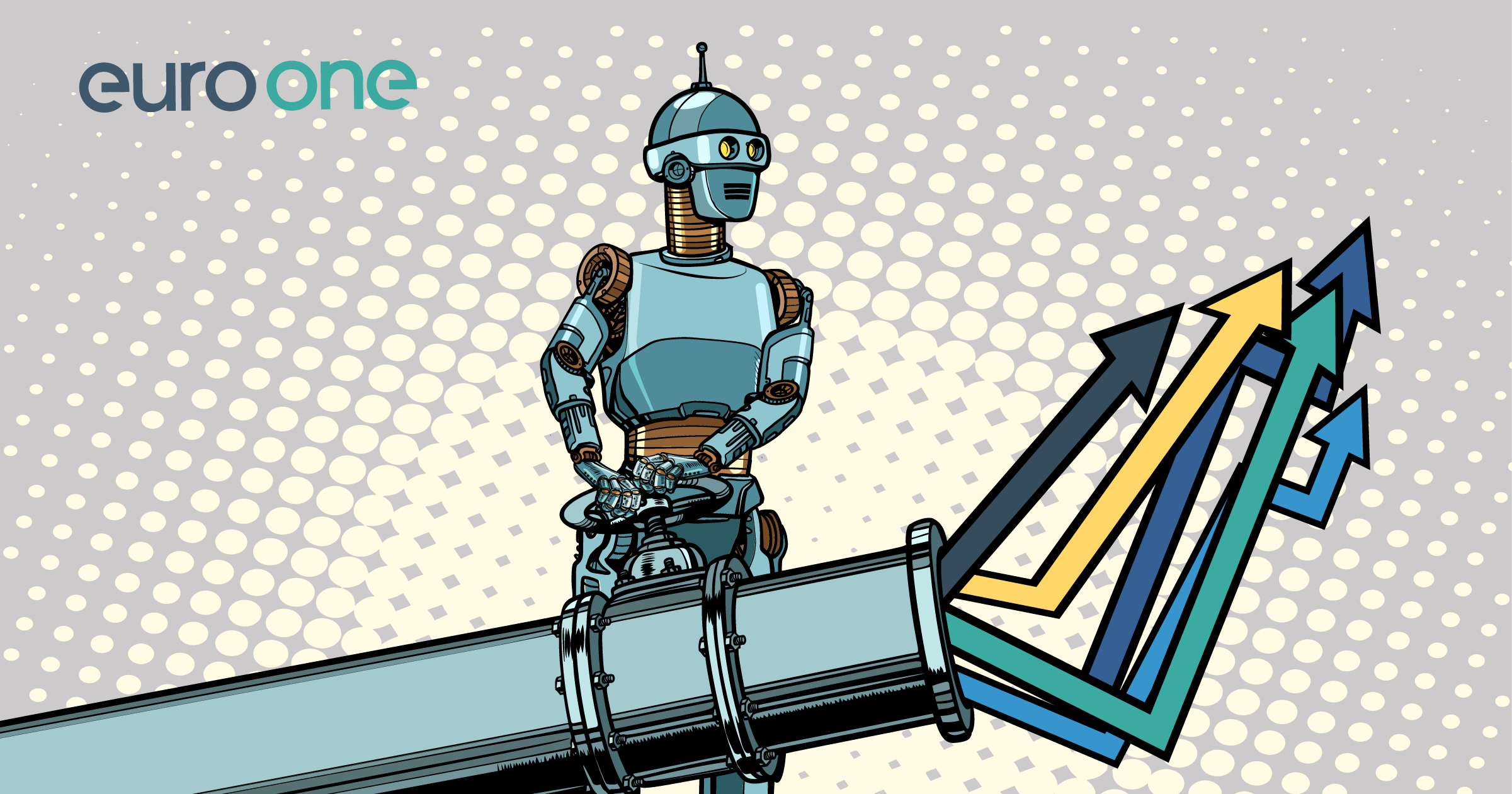
As cyber threats evolve, traditional network traffic analysis (NTA) tools that rely on fixed rules and signatures struggle to keep up. Today’s attackers use stealthy, dynamic methods—prompting a shift toward AI and ML-driven Network Detection and Response (NDR) solutions.
Limitations of Traditional NTA and the Emergence of AI/ML
Conventional NTA tools can’t keep pace with today’s threats due to their reliance on fixed rules. AI/ML offers a more dynamic, adaptable solution.
- Inability to detect unknown threats: Rule-based systems miss zero-days and new malware.
- High false positive rate: Too many alerts waste resources.
- Difficult to maintain and update: Manual rule updates are time-consuming.
- Limited contextual understanding: Hard to detect complex attack chains.
Key AI/ML Techniques in NDR Solutions
Modern NDR platforms apply a mix of learning models to spot threats effectively.
- Unsupervised Learning: Detects anomalies in unlabeled data (e.g., clustering).
- Supervised Learning: Learns from labeled traffic to identify known attacks.
- Deep Learning: Uses neural networks to spot subtle or hidden threats.
- Behavioral Analytics: Builds user/device profiles to flag unusual actions.
- Natural Language Processing (NLP): Helps analyze logs and threat intel.
Different Aspects of Network Data Analysis
AI/ML models work across multiple data types for deeper insights.
- Metadata: Analyzes IPs, ports, traffic size for unusual patterns.
- Full Packet Capture (FPC): Enables deep traffic inspection for hidden threats.
- Logs: Correlates system and device logs to track suspicious activities.
How AI/ML-Based NDR Systems Work
NDR systems follow a structured process, enabling intelligent threat detection.
- Data collection and pre-processing: Collects and normalizes network data.
- Feature Engineering: Extracts meaningful patterns and metrics.
- Training: Models learn normal behavior or known threats.
- Real-time Analysis: Scans traffic instantly for anomalies or matches.
- Alert Generation: Notifies security teams of suspicious activity.
- Response and Remediation: Some systems act automatically (e.g., isolate devices).
- Continuous Learning: Models improve over time based on new data.
Integration with Other Security Tools
NDR systems enhance and integrate with existing cybersecurity ecosystems.
- SIEM: Sends alerts for cross-event correlation.
- SOAR: Triggers automated responses.
- EDR: Complements endpoint monitoring with network insights.
- Threat Intelligence Platforms (TIP): Adds context to alerts via threat feeds.
Practical Examples of the Use of AI/ML in NDR
- Anomaly detection: Unusual spikes or unknown traffic sources.
- C2 detection: Finds covert command-and-control activity.
- Data leakage detection: Flags large or unexpected data transfers.
- Lateral movement: Detects attacker pivoting within the network.
- Zero-day behavior: Identifies system actions tied to new exploits.
Summary
AI and ML are critical for defending against today’s complex cyber threats. They enable faster detection, fewer false positives, and smarter responses—making them the foundation of next-generation NDR systems.
Read the full article on our International subsidiary’s website by clicking on the image.
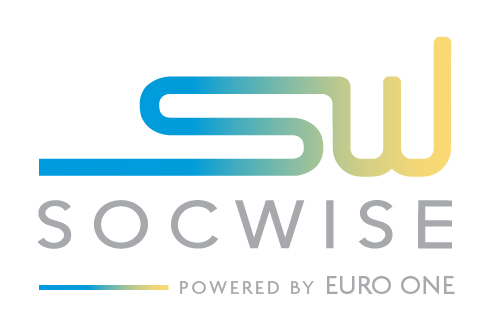